MLflow
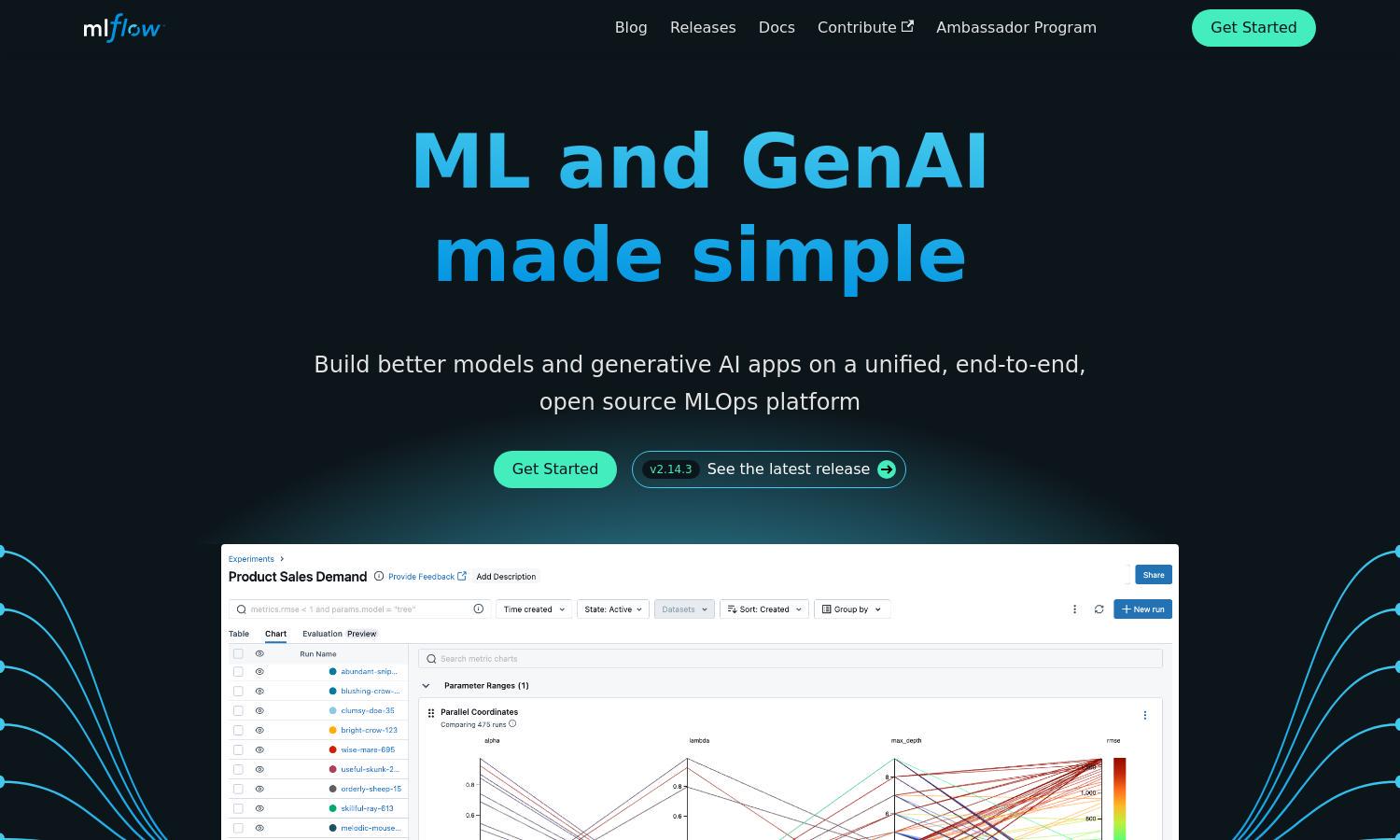
About MLflow
MLflow is an open-source platform tailored for data scientists and ML engineers, designed to streamline machine learning and generative AI projects. With its robust features like experiment tracking and model management, MLflow empowers users to efficiently develop, deploy, and optimize models, addressing real-world challenges in AI.
MLflow offers a free open-source model, while premium tiers include enhanced features and support. Users can upgrade for additional capabilities like advanced integrations and dedicated support, ensuring efficient management of ML projects. Explore MLflow’s unique value proposition and get the most out of your machine learning workflows.
MLflow boasts a user-friendly interface designed for seamless navigation. Its well-structured layout helps users access essential features such as experiment tracking and model deployment effortlessly. The intuitive design enhances the overall experience, ensuring even novice users can effectively manage their machine learning projects with MLflow.
How MLflow works
Users begin by setting up an MLflow account, allowing them to navigate its user-friendly interface. They can start tracking experiments by logging parameters and metrics, while accessing model management tools to store and version models. With straightforward deployment processes, users can easily host and scale their ML solutions using MLflow.
Key Features for MLflow
Experiment Tracking
MLflow's experiment tracking feature allows users to log, compare, and visualize their machine learning experiments effortlessly. This unique aspect showcases the platform's ability to enhance decision-making processes for data scientists, enabling informed adjustments to models and methodologies while ensuring optimal results.
Model Registry
MLflow's Model Registry provides a centralized repository for managing machine learning models and versions. By streamlining model transitions from development to production, this feature enhances collaboration and governance for teams, ensuring every model's lifecycle is efficiently managed throughout its usage.
Unified Deployment
MLflow offers unified deployment capabilities across various platforms, enabling users to deploy ML models seamlessly in different environments. This feature simplifies the deployment process, allowing users to focus on building high-quality models without worrying about the complexities of varied infrastructures.
You may also like:
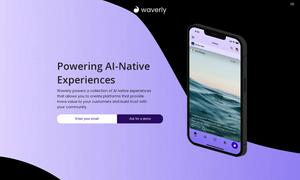
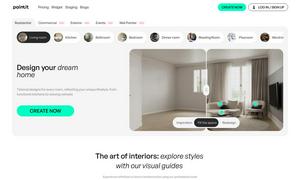